Labeled Metabolomics data analysis and integration with DIMet
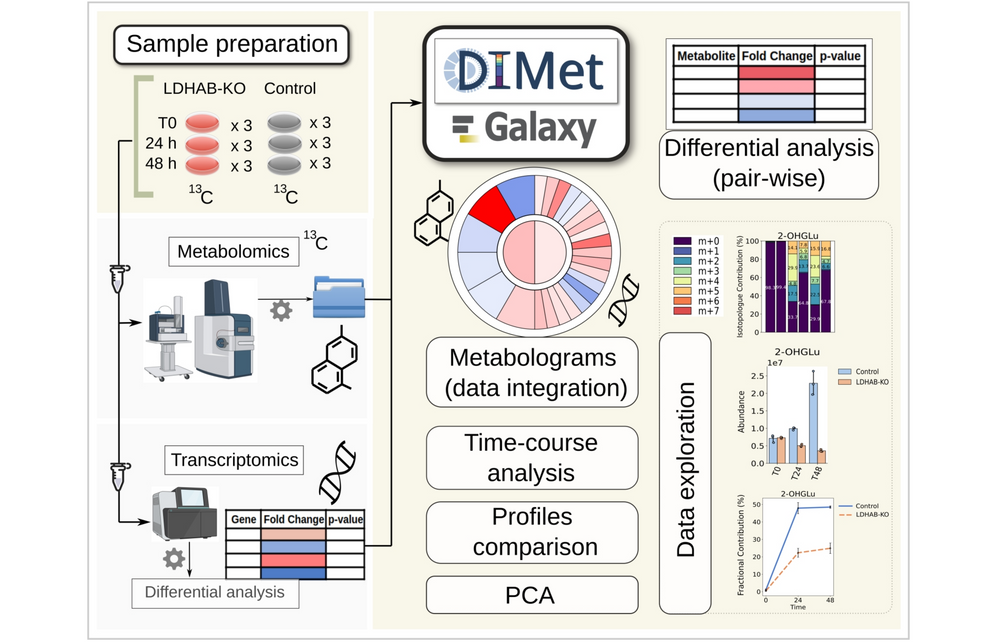
We’re excited to introduce DIMet (https://github.com/cbib/DIMet/wiki), a powerful tool for the differential analysis of isotope-labeled metabolomics data, developed by our team and recently published in Bioinformatics (Galvis J. et al., 2024; https://doi.org/10.1093/bioinformatics/btae282).
DIMet is designed specifically for isotope-labeled experiments, where stable isotopes (e.g., ¹³C) trace metabolic reactions by tracking their incorporation into metabolites. This tool accommodates the unique characteristics of labeled metabolomics data and offers a comprehensive suite of exploratory and statistical analyses, including uni-, bi-, and multivariate approaches. For preprocessing, it pairs seamlessly with TraceGroomer (https://github.com/cbib/TraceGroomer/wiki), supporting various input formats and normalization methods.
One of DIMet's standout features is its ability to integrate metabolomics with transcriptomics data through pathway-based metabolograms, providing an intuitive visualization of metabolic pathway perturbations. For example, in a study on lactate dehydrogenases (LDHA, LDHB) led by Thomas Daubon (EMBO Mol Med, Guyon J. et al., 2022), DIMet identified 21 differentially abundant or labeled metabolites in hypoxia-affected P3 cells (double-KO vs. control) and highlighted changes in Glycolysis, the TCA Cycle, and Gluconeogenesis pathways.
To ensure accessibility, DIMet and TraceGroomer are available on Galaxy Europe (https://usegalaxy.eu) and Workflow4Metabolomics (https://workflow4metabolomics.usegalaxy.fr), enabling seamless integration into other pipelines for downstream analysis of labeled metabolomics data.