Antimicrobial resistance prediction by machine learning approach
AI method for predicting the minimum inhibitory concentration (MIC) of several antibiotics for antimicrobial resistance (AMR)
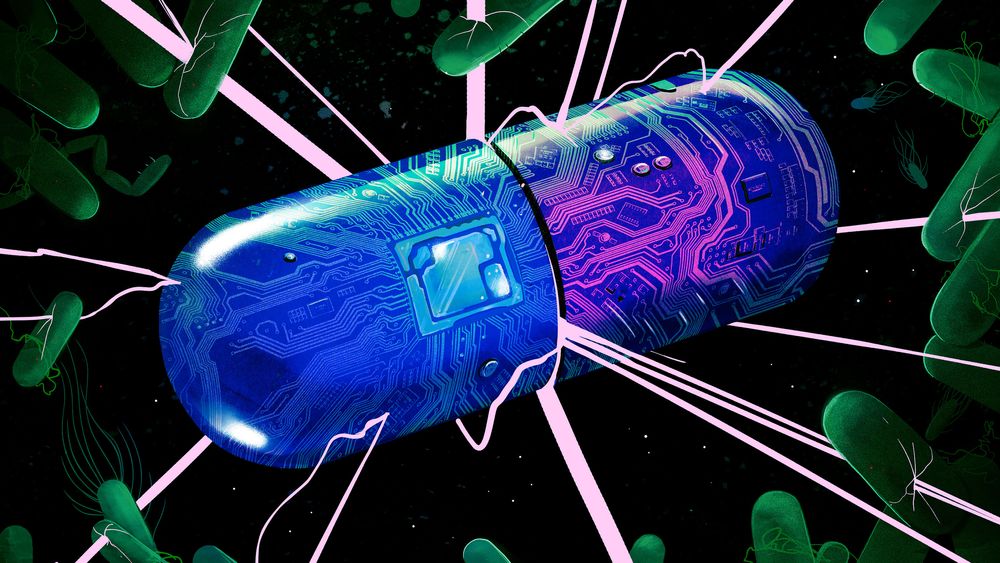
Anti-microbial resistance (AMR) has become a major public health concern due to the rapid emergence of multidrug-resistant bacteria, posing serious problems for the prevention and treatment of persistent infections.
In recent years, the significant increase in the generation of genomic data for a wide range of bacteria suggests that the development of algorithms that use genomic information to predict phenotypic variations such as AMR could be of major clinical importance, more reliable and efficient compared to traditional phenotyping, and could contribute to the discovery of previously unknown AMR pathways. We developed an AI method (ARSENAL) for predicting the minimum inhibitory concentration (MIC) of several antibiotics. This method is applied on the newly sequenced genomes of 1312 and 300 strains of Streptococcus pneumoniae and Pseudomonas aeruginosa, respectively.
ARSENAL relies on one hand on the sequence (k-mers), and on the other hand on the genome structure (in genes) and the gene orthology links between the strains of the same species (gene families) in order to enable functional interpretation of the most predictive features. The end-goal is to integrate the use of AI-based predictions in lab practice to streamline selection of treatments by accelerating the assessment of the level of resistance, provide insights for the generation of novel antibiotics, and assist in the surveillance of human and livestock AMR around the globe.