About us
Computational Biology and Bioinformatics is an interdisciplinary team at the CNRS institute in Bordeaux focused on multi-omics and imaging data integration. Our two main pillars are 1) Tackling real biological problems and 2) Combining different areas of technical expertise ...
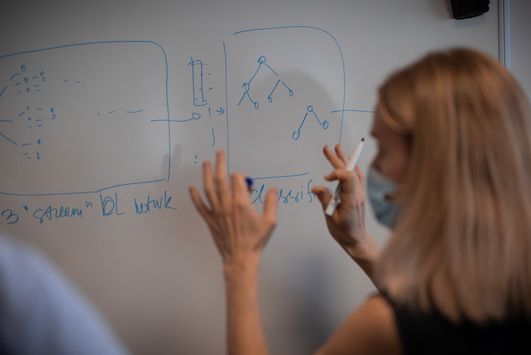
Computational Biology and Bioinformatics is an interdisciplinary team at the CNRS institute in Bordeaux focused on multi-omics and imaging data integration. The identity of our team is founded on two main pillars:
- We are interested in tackling real biological problems, with the main focus on human diseases. For this, we develop data-driven approaches leading us to introduce new concepts and methods in bioinformatics and computational biology.
- We take a pragmatic approach and combine different areas of technical expertise (algorithmics, artificial intelligence, data and network analysis) to tackle biological problems.
The central goal of the CB&B team is to advance research on the convergence between omics and microscopy imaging from the perspective of computational methods.
We are the reference scientific team for the Bioinformatics Core Facility at the University of Bordeaux, the CBiB.
Main Axes
Understanding sample heterogeneity
We are interested in the deconvolution of sequencing samples for the analysis of tumor heterogeneity and identification of clonal populations from bulk RNAseq or DNAseq tumor, and in the sequence deconvolution in the context of virome studies, between the host and different viruses.
Characterizing disease phenotype by modeling multi-omics and imaging data
This part of our research program is devoted to developing primary and integrative computational tools utilizing artificial intelligence, machine learning, and data mining methods. Applications include the study of the subtypes of neural cells involved in neuropathy, and of immune and tumoral cells responsible for tumor invasiveness.
Building predictive models of the phenotype based on multi-omics and imaging data
We are interested in linking our analyses of heterogeneous data with relevant phenotypic traits by building predictive models. In particular, we will pursue this avenue for the classification of cells (in application to glioblastoma, hepatocellular carcinoma and neuropathy) as well as for the prediction of antibiotic microbial resistance.
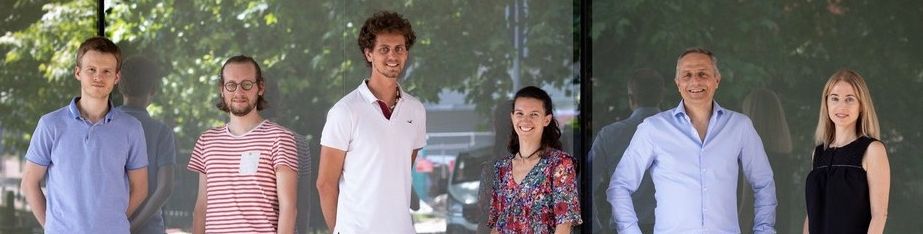
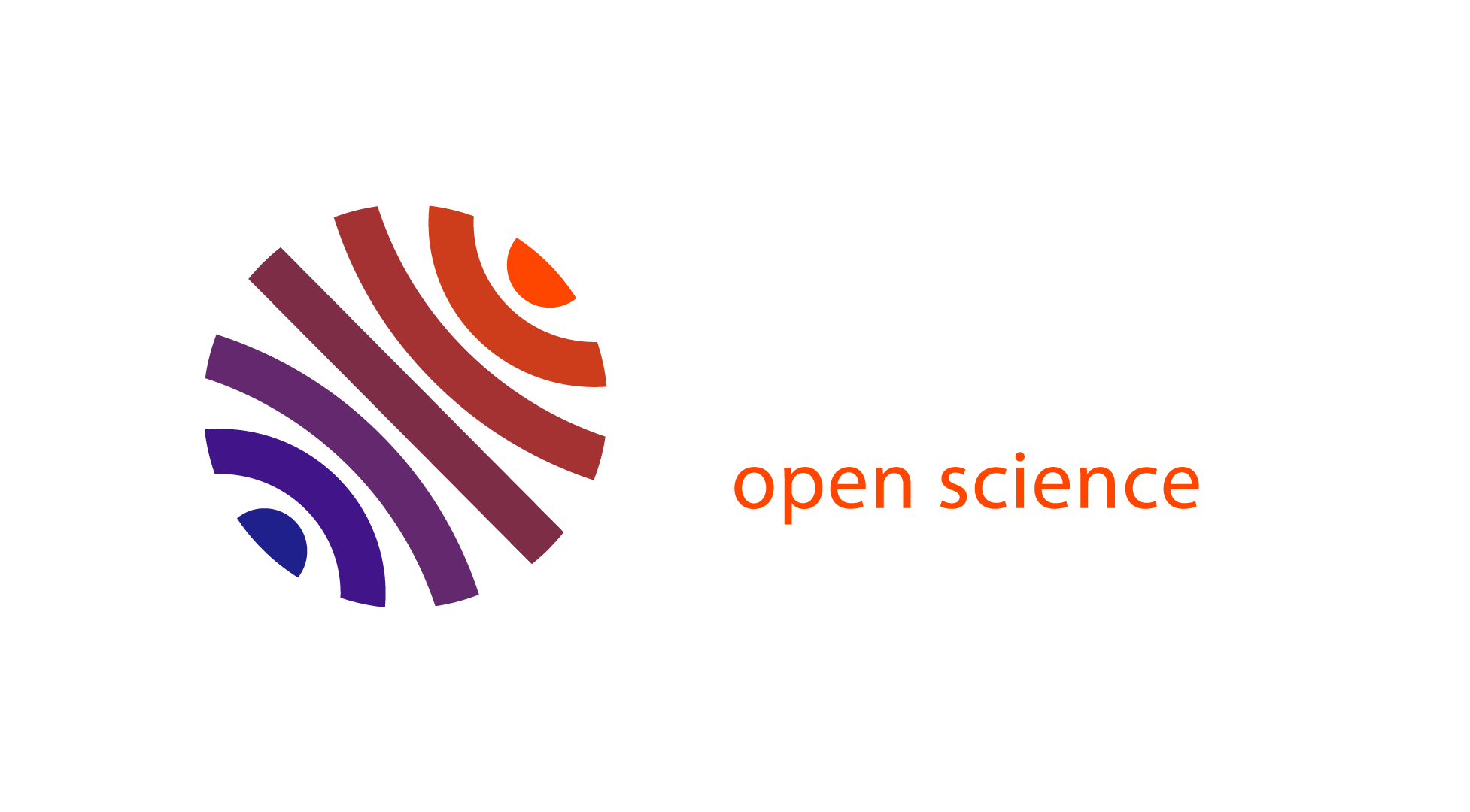
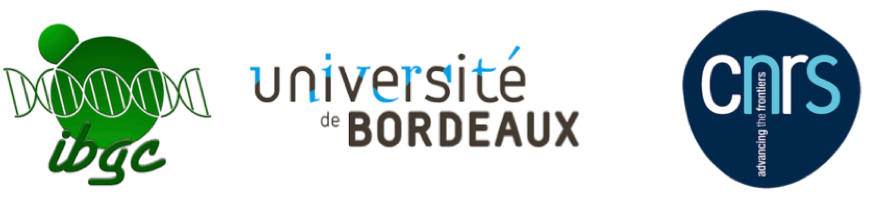