Unsupervised segmentation of nuclei in fluorescence microscopy images using Deep Learning
Development of unsupervised Deep Learning methods for segmentation of liver spheroids acquired with the soSPIM in 3D and prediction of temporal phenotypes of spheroids
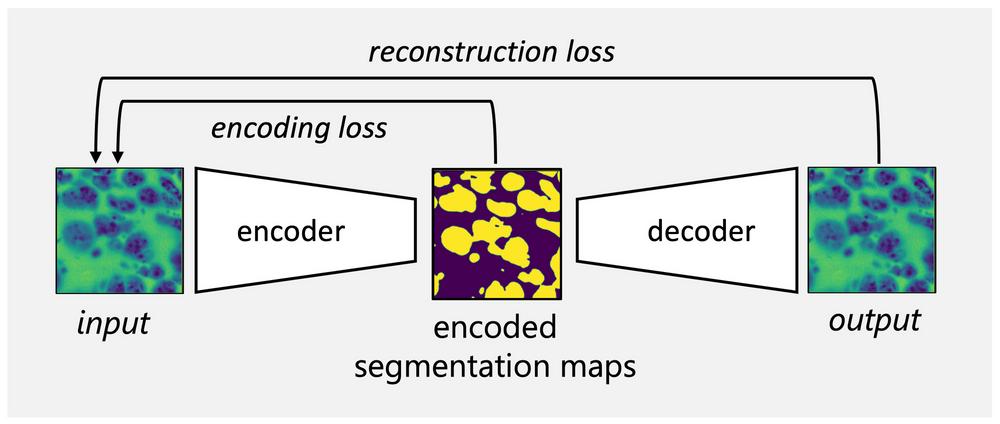
As current state-of-the-art segmentation algorithms rely on ground truth acquired from the manual image annotation, unsupervised learning algorithms have the potential to save time and effort otherwise dedicated to object labelling. We will build on previously established autoencoder networks such as W-Net [1] and focus on the design of loss functions that support the network in learning nuclei-specific features. The project aims to extend the method for three-dimensional images of liver spheroids acquired with the soSPIM (single-objective Selective Plane Illumination Microscopy) set-up [2] at the Interdisciplinary Institute for Neuroscience (IINS). In parallel, we are working in collaboration with IINS to establish a framework for the prediction of future temporal phenotypes in liver spheroids based on images acquired during the early stages of development.